As manufacturers move to adopt the technologies that address the challenges in today’s chaotic business environment, they are beginning to face a challenge many thought they would never have, too much data! The desire to digitally transform their business and drive towards the vision of Industry 4.0 has been facilitated by the wave of IIoT enabled devices on the factory floor that are generating a continual stream of manufacturing data.
These devices are the building blocks of the smart factory. The information they provide has enabled a raft of capabilities such as highly accurate predictive maintenance (PdM) tools that drastically reduce downtime by minimizing unplanned outages. The information from these devices can also be used to drive quality improvements, reduce energy usage, and enhance process performance. However, despite significant technology investments, businesses still are reporting anywhere from 50% to 70% of their digital transformation investments failing to deliver the expected benefits. The problem in many of these stalled transformation projects isn’t that there isn’t enough data but rather there is too much data.
The Problem with Viewing Data in a Silo
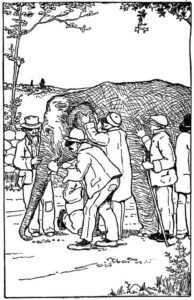
Many cultures have some variation of the parable of the “blind men and the elephant,” which is believed to have originated from India. The story is about six blind men who each inspect an elephant from a different perspective, coming to different conclusions as to what the animal must be. The first person, whose hand landed on the trunk, said, “This being is like a thick snake.” The next touched its ear and assumed it was a fan. The third person, whose hand was upon its leg, said, the elephant is a pillar-like tree trunk. The fourth blind man who placed his hand upon its side said the elephant,” is a wall. The fifth felt its tail and described it as a rope. The last felt its tusk, stating the elephant was made of bone, and smooth and like a spear.
In manufacturing, we often have the same problem. When data is viewed in isolation, it can be used to define a specific problem or assess a situation that sometimes can lead to inaccurate conclusions. When viewed only through the perspective of your role, it is often difficult to see without bias. Similarly, as the volume of data has grown with the explosion of digital sensors becoming the norm, it is becoming harder to focus on what data will give us the answers we are seeking while avoiding this bias.
In some cultures, the parable refers to men in a darkened room who are astounded when the lights come on and they can see the entire elephant. For manufacturers, the challenge in using the wealth of IIoT data is when it is used out of a broader context. The use of data by one part of the organization should not be viewed individually, based on the context of each person’s role.
The blind man grasping the trunk (snake) of the elephant might think the best way to contain the elephant would be to put it in a basket, just as a snake charmer might use. The blind man touching the tusk (spear) would think that simply storing it by leaning it against a wall would be the answer. In each case, a biased perspective leads to an inaccurate conclusion – this is exactly what can occur when looking at manufacturing data out of context.
Avoiding Decision Paralysis
Alternatively, when expanding your perspective and breaking out of your silo by looking at a broader segment of data, there is the risk of being overwhelmed with too much data. Today’s automobile can generate as much as 25 gigabytes of data every hour. The modern manufacturing plant can generate several orders of magnitude more data. Trying to make use of all the data when making decisions would likely lead to decision paralysis where data appears to provide conflicting guidance.
Having too much data has proven to be one of the bigger challenges with creating a truly autonomous vehicle – the inability to process information fast enough to make a decision that might result in no decision at all. In manufacturing, this can manifest itself in the classic case of a problem-solving meeting where everyone spends more time discussing whose data is correct instead of focusing on what the data is telling them.
This issue is frequently the result of not only having too much data but of having that data being collected and processed in multiple systems, each becoming their silo. One of the fundamental principles of any quality program is that everyone must be looking not only at the same data but using the same tools to analyze it. This is the only way to reach a consensus as to what the problem is and how it can be fixed.
As the volume of manufacturing data continues to explode, this issue will become even more pronounced unless the data is standardized and is available in context. By defining a manufacturing data architecture – ideally as part of your operations management platform – you can capture the greatest value from the ever-increasing amount of data that is coming from your manufacturing systems. This is a compelling case to digitalize all of your operations, to then have an associated context with all data collected, which is then made available to all employees to access and extract intelligence for decision support.